Big Image Data, Artificial Intelligence, and
The Future of Digital Pathology
Modern medicine is inconceivable without all imaging modalities available to radiologists, oncologists, cardiologists, pathologists and other clinicians. Computed tomography, magnetic resonance imaging, and ultrasound imaging are among the most commonly used imaging techniques. These technologies enable us to look inside the human body for diagnosis, treatment and monitoring purposes. Innovative technologies constantly emerging with the ultimate goal of increasing the quality of these images, among others the resolution and contrast.
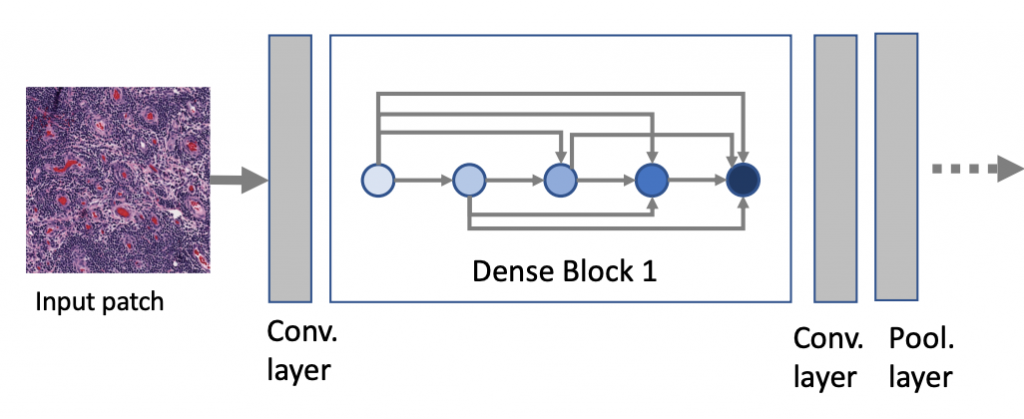
We are amid a transition from traditional pathology to digital pathology where scanners are replacing microscopes rapidly. Capturing the tissue characteristics in digital formats opens new horizons for diagnosis in medicine. On one hand, we will need to store thousands and thousands of specimens in large physical archives of glass samples. This will be a relief for many hospitals with limited space. On the other hand, acquiring an image from the specimen enables more systematic analysis, collaboration possibilities and, last but not least, the computer-aided diagnosis for pathology, arguably the final frontier of vision-based disease diagnosis.
KIMIA Lab has been founded with the specific mandate to explore knowledge mining in large medical image archives using advanced machine-learning algorithms. We use deep networks, supervised and unsupervised clustering methods, dimensionality reduction, and generative models along with cutting-edge computer vision algorithms to overcome the challenges of dealing with big image data in digital pathology.
KIMIA Lab, established on October 20, 2013 at the University of Waterloo, envisions conducting research at the forefront of big image data in archives of histopathology scans with the ultimate goal of extracting information that cannot only support a more speedy and accurate diagnosis and treatment of many diseases but also, and more significantly, establish new quality assurance based on mining of collective knowledge and wisdom.